Ability to Predict Melanoma Within 5 Years Using Registry Data and a Convolutional Neural Network: A Proof of Concept Study
DOI:
https://doi.org/10.2340/actadv.v102.2028Keywords:
area under curve, deep learning, epidemiologic methods, machine learning, melanoma, ROC curve, sensitivity and specificity, receiver-operating characteristic curveAbstract
Research relating to machine learning algorithms, including convolutional neural networks, has increased during the past 5 years. The aim of this pilot study was to investigate how accurately a convolutional neural network, trained on Swedish registry data, could perform in predicting cutaneous invasive and in situ melanoma (CMM) within 5 years. A cohort of 1,208,393 individuals was used. Registry data ranged from 4 July 2005 to 31 December 2011, predicting CMM between 1 January 2012 and 31 December 2016. A convolutional neural network with one-dimensional convolutions with respect to time was trained using healthcare databases and registers. The algorithm was trained on 23,886 individuals. Validation was performed on a holdout validation set including 6,000 individuals. After training and validation, the convolutional neural network was evaluated on a test set (1,000 individuals with an CMM occurring within 5 years and 5,000 without). The area under the receiver-operating characteristic curve was 0.59 (95% confidence interval (95% CI) 0.57–0.61). The point on the receiver-operating characteristic curve where sensitivity equalled specificity had a value of 56% (sensitivity 95% CI 53–60% and specificity 95% CI 55–58%). Albeit at an early stage, this pilot investigation demonstrates potential usefulness for machine learning algorithms in predicting melanoma risk.
Downloads
References
Saba L, Biswas M, Kuppili V, Cuadrado Godia E, Suri HS, Edla DR, et al. The present and future of deep learning in radiology. Eur J Radiol 2019; 114: 14-24.
https://doi.org/10.1016/j.ejrad.2019.02.038 DOI: https://doi.org/10.1016/j.ejrad.2019.02.038
Esteva A, Kuprel B, Novoa RA, Ko J, Swetter SM, Blau HM, et al. Dermatologist-level classification of skin cancer with deep neural networks. Nature 2017; 542: 115-118.
https://doi.org/10.1038/nature21056 DOI: https://doi.org/10.1038/nature21056
Haenssle HA, Fink C, Schneiderbauer R, Toberer F, Buhl T, Blum A, et al. Man against machine: diagnostic performance of a deep learning convolutional neural network for dermoscopic melanoma recognition in comparison to 58 dermatologists. Ann Oncol 2018; 29: 1836-1842. DOI: https://doi.org/10.1093/annonc/mdy520
https://doi.org/10.1093/annonc/mdy166 DOI: https://doi.org/10.1093/annonc/mdy166
Han SS, Park GH, Lim W, Kim MS, Na JI, Park I, et al. Deep neural networks show an equivalent and often superior performance to dermatologists in onychomycosis diagnosis: Automatic construction of onychomycosis datasets by region-based convolutional deep neural network. Plos One 2018; 13: e0191493.
https://doi.org/10.1371/journal.pone.0191493 DOI: https://doi.org/10.1371/journal.pone.0191493
Chu YS, An HG, Oh BH, Yang S. Artificial intelligence in cutaneous oncology. Front Med (Lausanne) 2020; 7: 318.
https://doi.org/10.3389/fmed.2020.00318 DOI: https://doi.org/10.3389/fmed.2020.00318
Wang S, Yang DM, Rong R, Zhan X, Xiao G. Pathology image analysis using segmentation deep learning algorithms. Am J Pathol 2019; 189: 1686-1698.
https://doi.org/10.1016/j.ajpath.2019.05.007 DOI: https://doi.org/10.1016/j.ajpath.2019.05.007
Cheng Y, Wang F, Zhang P, Hu J. Risk prediction with electronic health records: a deep learning approach. Proceedings of the 2016 SIAM International Conference on Data Mining: SIAM, 2016: p. 432-440.
https://doi.org/10.1137/1.9781611974348.49 DOI: https://doi.org/10.1137/1.9781611974348.49
Du AX, Emam S, Gniadecki R. Review of machine learning in predicting dermatological outcomes. Front Med (Lausanne) 2020; 7: 266.
https://doi.org/10.3389/fmed.2020.00266 DOI: https://doi.org/10.3389/fmed.2020.00266
Whiteman DC, Green AC, Olsen CM. The growing burden of invasive melanoma: projections of incidence rates and numbers of new cases in six susceptible populations through 2031. J Invest Dermatol 2016; 136: 1161-1171.
https://doi.org/10.1016/j.jid.2016.01.035 DOI: https://doi.org/10.1016/j.jid.2016.01.035
Buja A, Sartor G, Scioni M, Vecchiato A, Bolzan M, Rebba V, et al. Estimation of direct melanoma-related costs by disease stage and by phase of diagnosis and treatment according to clinical guidelines. Acta Derm Venereol 2018; 98: 218-224.
https://doi.org/10.2340/00015555-2830 DOI: https://doi.org/10.2340/00015555-2830
Tinghog G, Carlsson P, Synnerstad I, Rosdahl I. Societal cost of skin cancer in Sweden in 2005. Acta Derm Venereol 2008; 88: 467-473.
https://doi.org/10.2340/00015555-0523 DOI: https://doi.org/10.2340/00015555-0523
Giannopoulos F, Gillstedt M, Laskowski M, Bruun Kristensen K, Polesie S. Methotrexate use for patients with psoriasis and risk of cutaneous squamous cell carcinoma: a nested case-control study. Acta Derm Venereol 2021; 101: adv00365.
https://doi.org/10.2340/00015555-3725 DOI: https://doi.org/10.2340/00015555-3725
Polesie S, Gillstedt M, Paoli J, Osmancevic A. Methotrexate treatment for patients with psoriasis and risk of cutaneous melanoma: a nested case-control study. Br J Dermatol 2020; 183: 684-691.
https://doi.org/10.1111/bjd.18887 DOI: https://doi.org/10.1111/bjd.18887
Statistics Sweden, Population Statistics. [accessed 2021 Dec 9]. Available from: https://www.statistikdatabasen.scb.se/pxweb/en/ssd/START__BE__BE0101__BE0101A/BefolkningR1860N/.
Wang HH, Wang YH, Liang CW, Li YC. Assessment of deep learning using nonimaging information and sequential medical records to develop a prediction model for nonmelanoma skin cancer. JAMA Dermatol 2019; 155: 1277-1283.
https://doi.org/10.1001/jamadermatol.2019.2335 DOI: https://doi.org/10.1001/jamadermatol.2019.2335
Vivot A, Gregory J, Porcher R. Application of basic epidemiologic principles and electronic health records in a deep learning prediction model. JAMA Dermatol 2020; 156: 472-473.
https://doi.org/10.1001/jamadermatol.2019.4919 DOI: https://doi.org/10.1001/jamadermatol.2019.4919
Cho SI, Lee D, Jo SJ. Application of basic epidemiologic principles and electronic health records in a deep learning prediction model. JAMA Dermatol 2020; 156: 473-474.
https://doi.org/10.1001/jamadermatol.2019.4922 DOI: https://doi.org/10.1001/jamadermatol.2019.4922
Richter AN, Khoshgoftaar TM. Efficient learning from big data for cancer risk modeling: a case study with melanoma. Comput Biol Med 2019; 110: 29-39.
https://doi.org/10.1016/j.compbiomed.2019.04.039 DOI: https://doi.org/10.1016/j.compbiomed.2019.04.039
Collins GS, Moons KGM. Reporting of artificial intelligence prediction models. Lancet 2019; 393: 1577-1579.
https://doi.org/10.1016/S0140-6736(19)30037-6 DOI: https://doi.org/10.1016/S0140-6736(19)30037-6
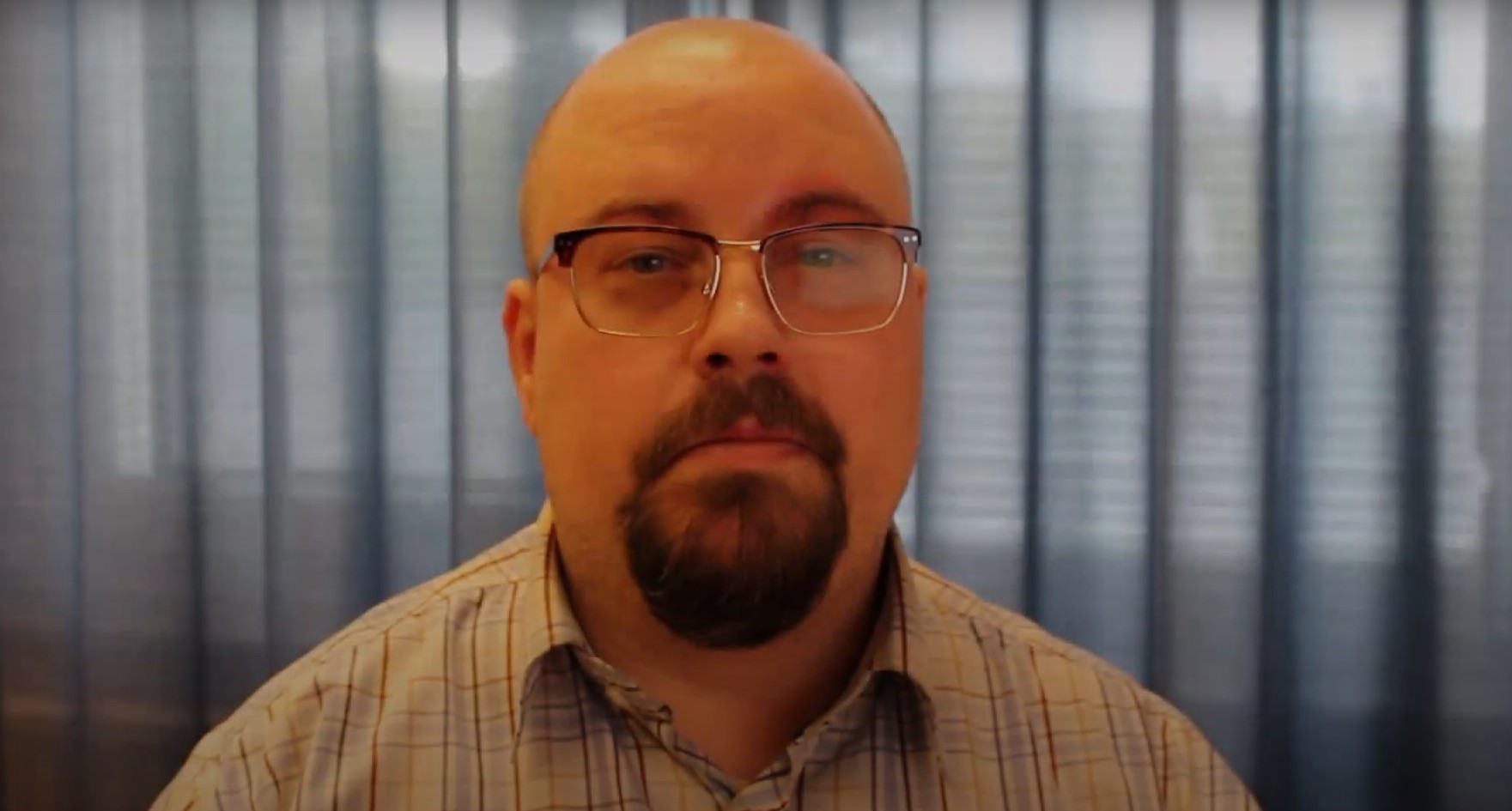
Downloads
Additional Files
Published
How to Cite
Issue
Section
Categories
License
Copyright (c) 2022 Martin Gillstedt, Sam Polesie

This work is licensed under a Creative Commons Attribution-NonCommercial 4.0 International License.
All digitalized ActaDV contents is available freely online. The Society for Publication of Acta Dermato-Venereologica owns the copyright for all material published until volume 88 (2008) and as from volume 89 (2009) the journal has been published fully Open Access, meaning the authors retain copyright to their work.
Unless otherwise specified, all Open Access articles are published under CC-BY-NC licences, allowing third parties to copy and redistribute the material in any medium or format and to remix, transform, and build upon the material for non-commercial purposes, provided proper attribution to the original work.